- Security
- AI
AI and digital product security: a symbiotic relationship
January 31 — 2025
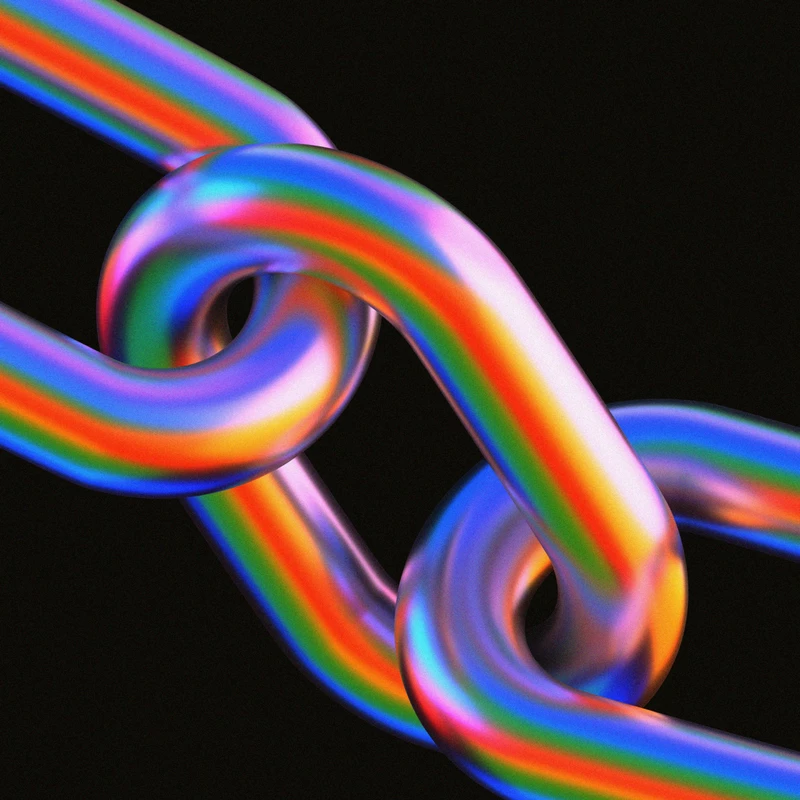
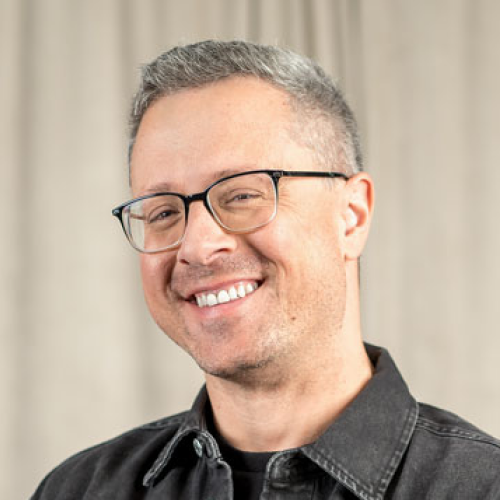
As artificial intelligence continues to reshape industries and push the boundaries of technology, the convergence of AI and cybersecurity — especially in application security — has emerged as both a breakthrough opportunity and a critical concern. Let's dive into the dynamic relationship between AI and application security, examining how they elevate each other while introducing new challenges to the landscape.
AI: the double-edged sword of modern cybersecurity
The swift evolution of AI has established it as both a powerful ally and a formidable opponent in cybersecurity. On one side, AI-driven solutions are revolutionizing our threat detection and mitigation capabilities. Machine learning algorithms excel at processing vast amounts of data to spot cyber attack patterns, leading to quicker and more effective responses.
For instance, AI excels at automatically detecting unusual application behaviors, identifying potential security breaches that human analysts might miss. This capability proves essential in defending against sophisticated threats that employ subtle techniques to breach systems.
Yet, cybercriminals are equally quick to harness AI's power to enhance their attacks. They're using AI to generate more sophisticated phishing emails, streamline vulnerability discovery in applications, and develop adaptive malware that evades detection systems. This situation has sparked a cyber arms race, with both defensive and offensive teams constantly advancing their AI capabilities.
Language models (LLMs): a new internal security risk
The rise of large language models (LLMs) presents a unique challenge in application security. While these models excel at tasks like code generation and customer support, they bring significant risks to the table. Bad actors can potentially exploit LLMs to generate vulnerable code or manipulate the AI systems into exposing sensitive information.
Moreover, LLMs can inadvertently serve as conduits for data leaks. Without proper security measures, these models might disclose confidential information learned during training. This vulnerability highlights critical concerns about data privacy and emphasizes the need for robust security protocols when implementing AI models within applications.
Enhancing application security through AI
While AI presents certain risks, it remains a powerful tool for strengthening application security. Organizations integrating AI into their security protocols can benefit from:
- Proactive threat detection: AI systems continuously monitor applications, identifying and detecting suspicious activities and potential threats before they escalate into major security incidents.
- Automated incident response: AI enables rapid threat mitigation through automated responses, including system isolation and traffic filtering to minimize attack surfaces.
- Advanced vulnerability management: Machine learning algorithms perform code analysis to detect security vulnerabilities, providing development teams with remediation recommendations and preventive measures.
- User behavior analytics (UBA): AI establishes behavioral baselines and identifies anomalous patterns that may indicate account compromise or insider threats.
Navigating AI-Cybersecurity integration challenges
The convergence of AI and application security presents several critical challenges:
AI model manipulation
Threat actors can execute adversarial attacks by feeding malicious inputs designed to compromise AI models, potentially undermining AI-powered security controls.
Data integrity and algorithmic bias
AI security systems require pristine training data. Flawed or biased datasets can compromise security effectiveness and trigger false positives, ultimately undermining confidence in AI-driven solutions.
Regulatory framework compliance
AI systems processing sensitive data must adhere to stringent regulations like Bill 25, GDPR, and HIPAA, demanding comprehensive data governance protocols.
Ethics and privacy concerns
AI-powered surveillance and behavior analytics raise significant privacy and consent issues, particularly in contexts like analyzing customer emotions to improve marketing strategies, employee monitoring, or public facial recognition deployment without explicit user consent.
Advancing the future: collaboration and innovation
To maximize AI's potential in application security, organizations must foster integration between cybersecurity experts, AI developers, and key stakeholders. This strategic framework includes:
- Developing secure AI models: Incorporating security-first principles throughout the AI development lifecycle. This includes implementing robust adversarial defense mechanisms, conducting comprehensive threat modeling, and enforcing strict data sanitization protocols while maintaining privacy-by-design principles.
- Continuous learning and adaptation: Maintaining AI system resilience through continuous model monitoring and regular updates to adapt to emerging attack vectors.
- Education and awareness: Training security professionals in AI technologies while ensuring AI developers understand security best practices.
- Research investment: Supporting initiatives that explore advanced AI applications in cybersecurity, including defenses against AI-powered attacks.
The symbiotic relationship between AI and application security continues to redefine modern cybersecurity. While AI brings new vulnerabilities to the surface, it simultaneously provides unprecedented capabilities to strengthen our defenses against evolving cyber threats. By embracing this powerful synergy and effectively addressing its inherent challenges, organizations can bolster their security posture and confidently navigate today's complex digital landscape.